About me
To start off, a little bit about me: I completed my PhD in Statistics in June 2020 at Stanford University, where I was lucky to be supervised by Professor Susan Holmes and where I had the chance to work with Prof. Jure Leskovec. Prior to Stanford, I studied Applied Mathematics and Engineering at Ecole Polytechnique (France), where I received an M.S and B.S equivalent.
As of August 2020, I am an Assistant Professor in the Department of Statistics at the University of Chicago.
• If you would like to be a postdoctoral fellow in the group, please send me an email at cdonnat [at] uchicago.edu including your interests and CV.
• If you are a UChicago PhD/Masters or Undergrad student interested in joining the group, please send me an email including your interests, CV, and transcript.
• For others not currently at the University, we apologize if we may not have the bandwidth to respond.
Research Interests
My research interests lie in the analysis of patterns and the quantification of uncertainty in high-dimensional datasets, and in particular, graphs and networks, geared towards biomedical applications.
Indeed, from brain connectomics to cybersecurity, graphs appear as an indispensable paradigm for studying complex relationships between entities. Yet, this formalism deviates from its traditional Euclidean counterpart in two essential ways: (1) Data points are connected and can therefore no longer be considered as independent. Rather, inference on graphs should be guided by their topological structure and leverage relevant edge, node and neighbourhood information; (2) Graph data is irregular: there exists no natural ordering of the nodes, no reference point, nor homogeneity in nodes’ topological roles (degree distribution, betweenness centrality, etc.). These properties imply that graph data is not amenable to analysis through standard Machine Learning (ML) and Statistics methods — and consequently offers a challenging canvas for the re-adaptation of these methods to the graph setting.
In this context, from the methods perspective, the research directions that I am currently investigating include:
- 1 - Graph Neural Networks. As the direct extension of the deep neural network machinery to graph data, GNNs have recently gained an important amount of traction. Yet, despite GNNs’ success on reference datasets in the academic community, both their properties and limitations remain ill-understood. Motivated by the necessity to shift GNNs from a “black-box” model to an actionable ML method that can be explained, trusted and relied upon in practical settings, we currently focus on the analysis, improvement of GNNs and graph algorithms at large, as well as their practical application.
Find out more about our GNN research. - 2- Network Inference: While networks offer an attractive formalism to study relational data, in many applications, the raw data has to be substantially processed to infer the graph structure before any network analysis can be achieved. In this setting, network inference is thus a crucial and indispensable component of the "graph analysis" pipeline, and on the quality of which depends any downstream analysis. As a result, we are interested in developping algorithms ablet to infer interactions and associations betweem agents in a network, as well as defining confidence levels around the inferred network structure.
Find out more about our Network Inference research. - 3- Latent Variable Models and Variational Inference: Finally, I am also extremely interested in Bayesian and Probabilistic Graphical models (PGMs). More specifically, I am interested in topics ranging from PGM inference and variational inference.
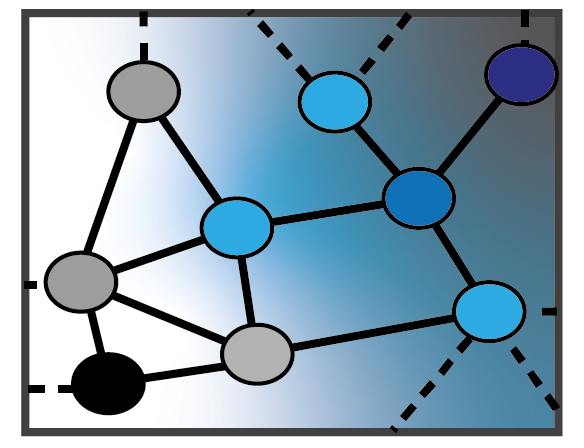
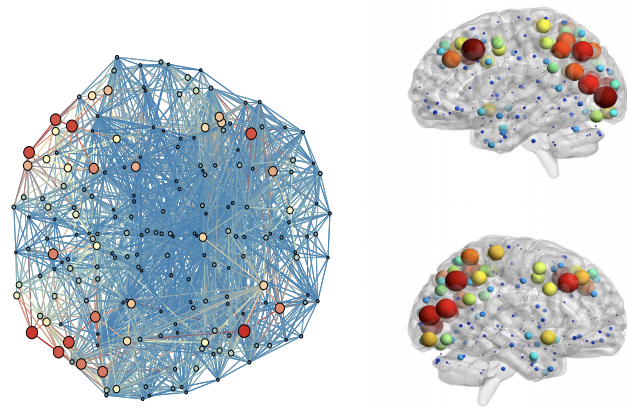
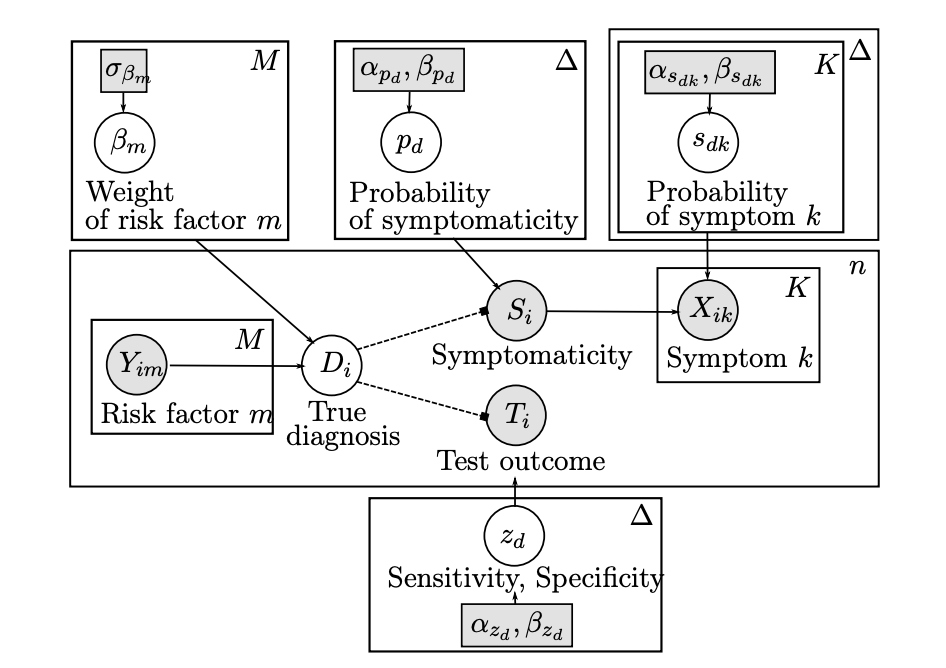
From the applications perspective, I am extremely interested in the potential of graphs and probabilistic models to biomedical problems. I have in the past and am currently continuing on working on biomedical, neuroscience as well as COVID-19 related applications. I am also part of a collaboration working on the reconstruction of protein shape from cryoEM images.